Blog /
Data Hallucinations and The Impact of AI Sustainability
Data Hallucinations and The Impact of AI Sustainability
This featured blog post was provided by our partners at Contextere.
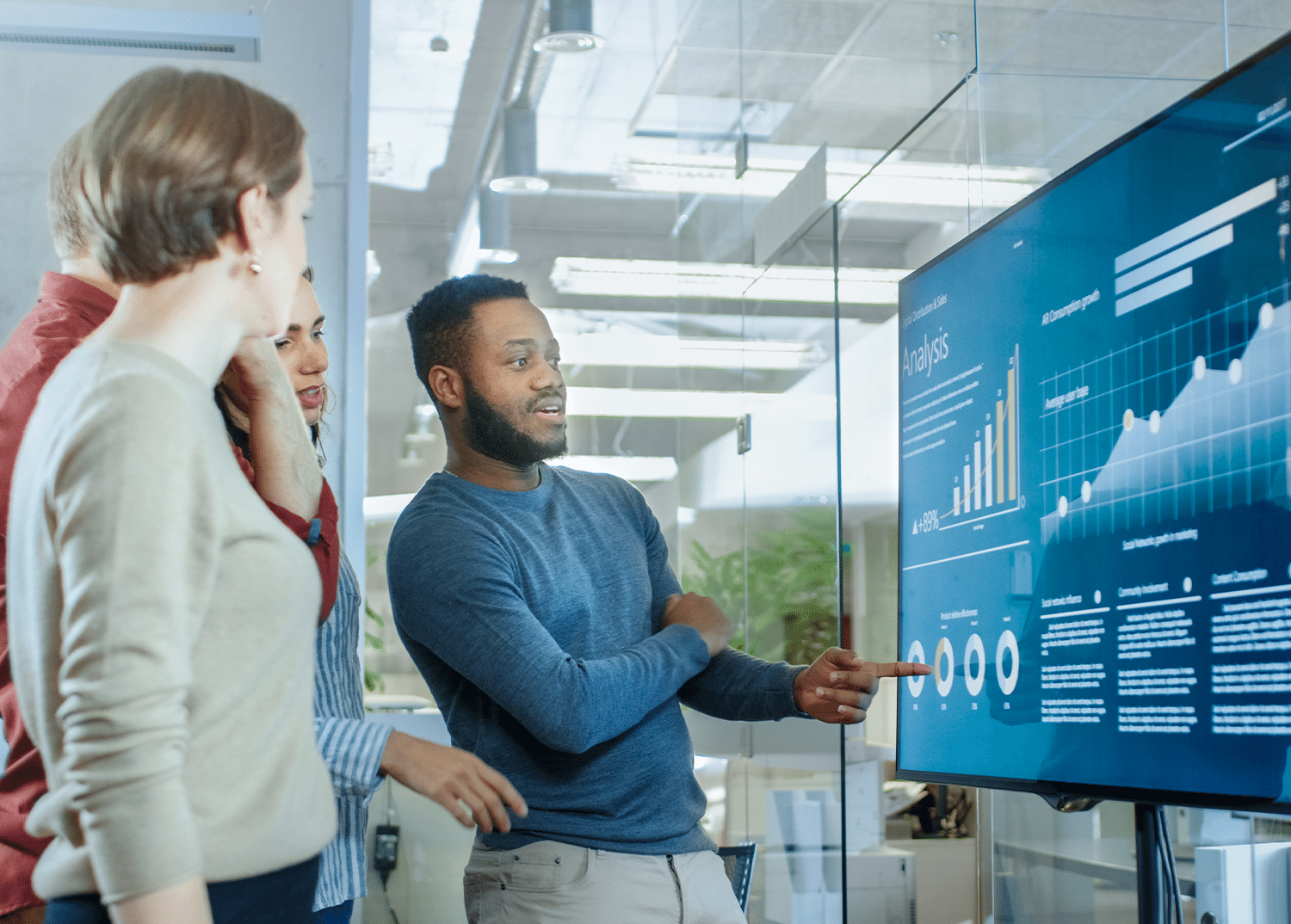
This blog was provided by our partners at Contextere.
Recently, there has been a growing concern surrounding the sustainability of AI technologies. Traditional large language models (LLMs), while powerful, come with significant computational demands. These demands translate into high energy consumption, contributing to an ever-growing carbon footprint. By moving towards smaller language and data models, we can achieve similar or superior outcomes with a fraction of the computational resources. In addition to the environmental benefits, this approach also has practical advantages, as it reduces costs and makes AI more accessible to organizations with fewer resources. The push towards smaller models represents a significant step in this direction, aiming to reduce emissions while improving accuracy and efficiency. As the urgency of addressing climate change becomes increasingly severe, the AI community is being called upon to reconsider its approach to sustainability. This shift is not just a technical evolution but a necessary move to align AI development with broader environmental and ethical goals.
One of the key challenges with traditional AI models is data hallucination, a phenomenon in which the model produces information that lacks verifiable sources. This leads to incorrect or misleading outputs which can have serious consequences in mission-critical environments with strong regulatory and safety requirements. Retrieval-Augmented Generation (RAG) AI addresses this issue through real-time retrieval of reliable information from knowledge sources, ensuring that the generated outputs are not only factually accurate but also verifiable through sources. MADISON, Contextere’s Insight Engine, utilizes RAG AI to optimize and ensure veracity in all user interactions. This technology ensures that workers and decision-makers are equipped with accurate, actionable insights, allowing them to do their jobs better the first time. By improving the quality of information available to workers, RAG AI enhances productivity, eliminates guesswork, and reduces the potential for costly errors.
AI development does not happen in a vacuum. To build technology that is both effective and sustainable, collaboration with industry is essential. Feedback from those on the front lines—whether in manufacturing, healthcare, or any other sector—provides invaluable insights that drive the refinement of AI models. This collaborative approach ensures that AI technologies are more aligned with industry needs and also more sustainable in their application. The more we engage with industry stakeholders, the better we can tailor AI solutions to real-world challenges, reducing waste, improving efficiency, and ensuring that AI development progresses in a direction that benefits business and fulfills ethical guidelines. The shift towards sustainability in AI is not just a trend—it’s an essential and imperative evolution that will shape the next generation of technological innovation. By prioritizing these aspects, we can create AI systems that are not only more efficient and accurate but also more responsible stewards of our planet’s resources.
Other Blogs
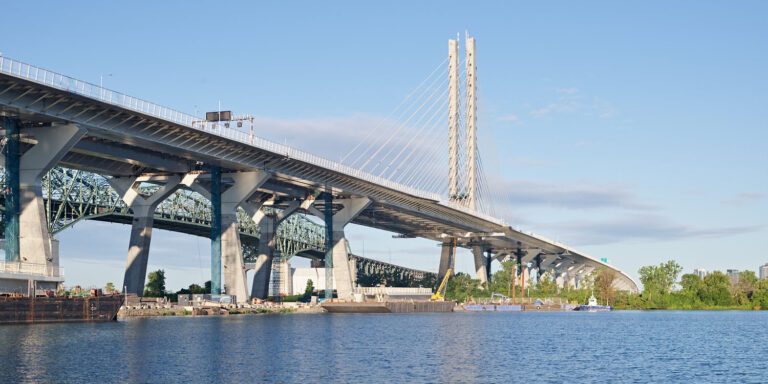
Navigating Net Zero
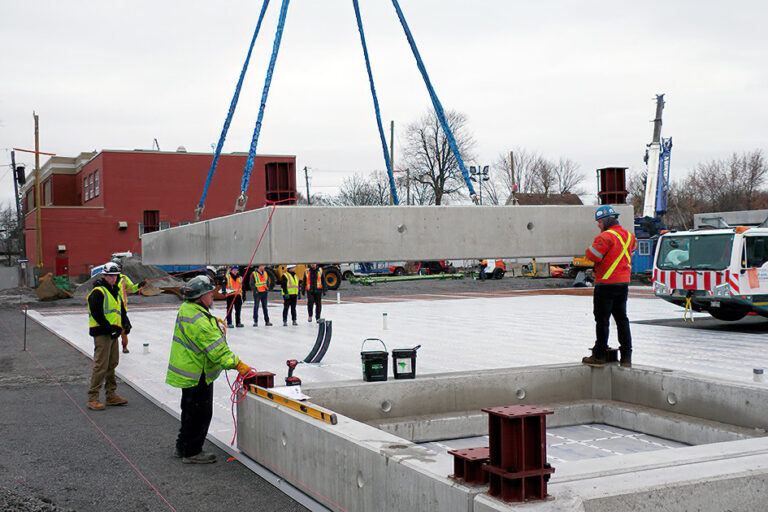
Lodestar Structures: Revolutionizing Community Infrastructure
